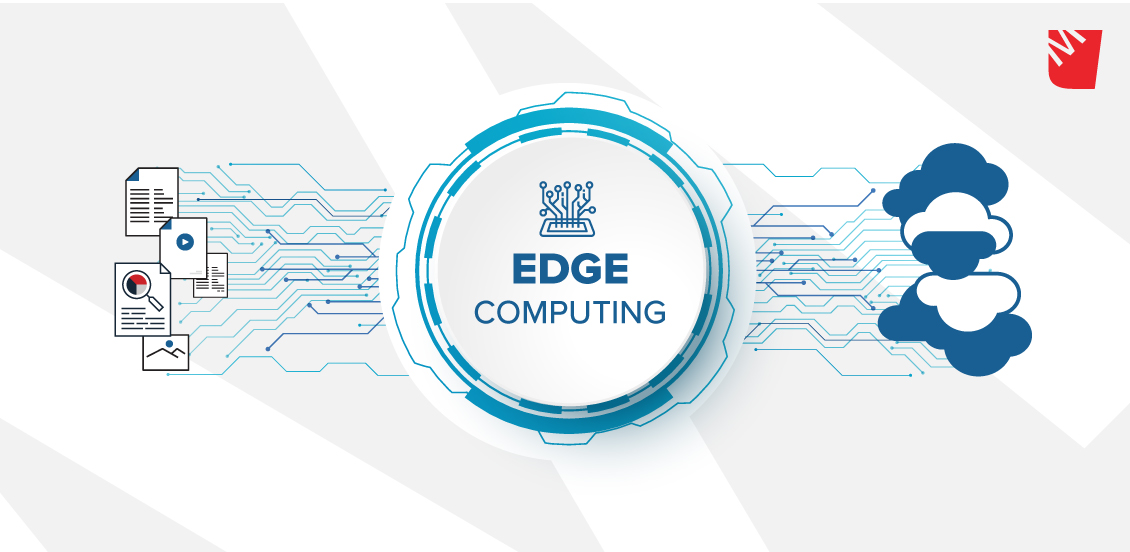
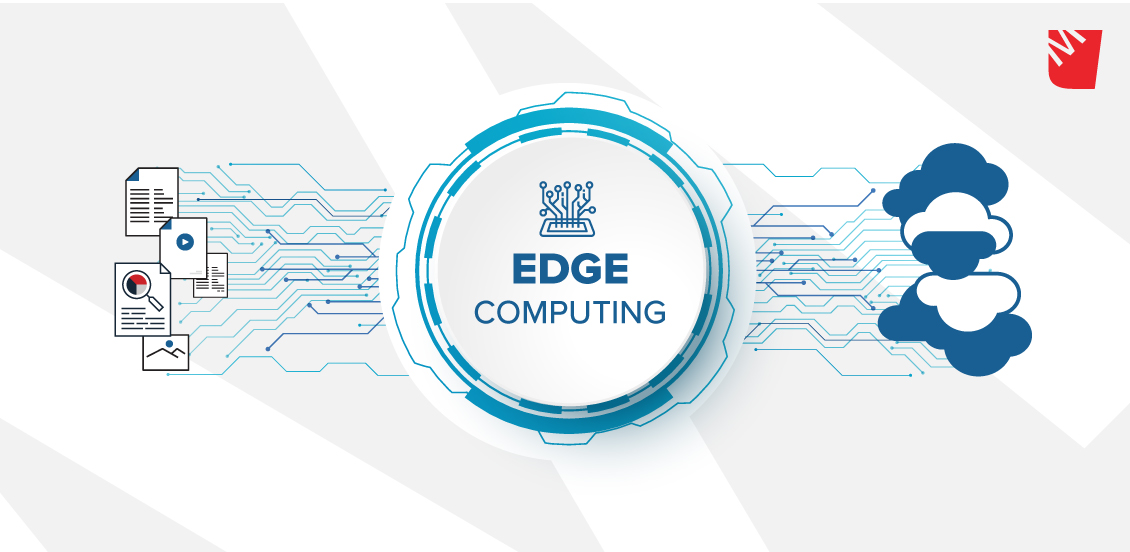
Edge Computing – A Building Block for Smart Applications of the Future
The information technology is changing every aspect of our life day by day. Edge Computing is a new computing paradigm in this respect which is foreseen to be a part of this change in addition to the Internet of Things (IoT), Machine Learning (ML) and Big Data Analytics.
Edge Computing aims to move computing from distant cloud environments to the edges of the networks. Hence, “smart” applications do not need to send data all the way to the cloud and get rid of waiting for the results to travel the long way back.
Mahadev Satyanarayanan, who is considered to be the “father” of Edge Computing, explains the birth of Edge Computing in his article [1]. Accordingly, the resource limitations of mobile devices required a resource-rich platform to process the data and perform computations on behalf of such devices. Cloud computing emerged as a solution to this problem, which offered significantly better resources (e.g., processing, memory and storage resources) for the computation need of mobile applications. However, gathering all the computation resources in a distant cloud environment started to cause issues for applications that are latency sensitive and bandwidth hungry. The underlying reason is that packets have to travel through several routers managed by Internet Service Providers (ISPs), operating at varying tiers. All these routers signify increase on the Round-Trip Times (RTTs) that latency-sensitive applications face. In addition to this, end-to-end routing path delays can change very dynamically due to ISPs and network conditions (Medianova R&D Center proposed a research paper on this issue to IEEE ISNCC 2019). What is more, as billions of IoT devices are expected to generate and transfer data to the cloud environments, it will be inevitable to face bandwidth bottlenecks. Therefore, distant cloud environments cannot be a solution for latency-sensitive and bandwidth-hungry applications. Edge Computing was born as a solution to this problem, that brings cloud resources to the edges of the networks, hence applications do not suffer from high RTTs and bandwidth bottlenecks. In Edge Computing, we move computation logic to the data, not the data to the computation logic [15]. The following figure shows our transition from Cloud Computing to Edge Computing where Edge Computing promises a huge latency gain for latency sensitive applications.
The transition from Cloud Computing to Edge Computing
In the concept of moving the computation resources from cloud to edge, cloudlet, fog and mobile edge computing technologies emerged [2,3]. In this respect, mobile edge computing promises to bring computing resources to the base stations of cellular networks. Hence cellular devices within the Radio Access Network (RAN) can easily offload computation and storage jobs to the Mobile Edge Computing nodes. Fog computing promises to bring computation resources to geographical regions by means of fog nodes, which can be routers, switches, access points, IoT gateways, and set-top boxes. Cloudlet computing refers to the computing paradigm in which latency sensitive and compute-intensive applications run on servers that are located within the local area networks. They can be called as the data center in a box [3], which promises small-scale datacenter resources to the Edge Computing users.
Several applications can benefit from Edge Computing. Among these artificial intelligence models [15], augmented reality applications, video analytics, IoT, location services and data caching can be given as the major applications. Many studies were proposed as Edge Computing use-cases in the literature too. In this respect, smart traffic light and smart wind farms [4], emergency alert service [5], fall detection [6], smart-parking [7] and lane changing in vehicular networks [8] were proposed as the fog computing applications. Mobile Edge Computing use-cases were presented in the form of proactive caching of website contents [9] and resource virtualization using containers [10]. In terms of Cloudlet Computing, on vehicle video gaming, road and traffic conditions monitoring [11], pre-processing of crowdsourcing data [12] and video analytics at the edge [13] were suggested. As a general Edge Computing solution providing the real-time analytics service using the Edge Computing capabilities was also proposed [14].
In order to create an ecosystem with standardized and open mechanisms for Edge Computing applications, a number of standardization organizations, consortiums, and initiatives have been joining forces. The IETF, ETSI, Open Edge Computing Initiative, Industrial Internet Consortium, and OpenFog Consortiums can be counted as examples of such initiative attempts.
References
1. Satyanarayanan, M. (2019). How we created edge computing. Nature Electronics, 2(1), 42-42. doi:10.1038/s41928-018-0194-x 2. Hassan, N., Gillani, S., Ahmed, E. Yaqoob, I. and Imran, M. (2018). The Role of Edge Computing in Internet of Things. IEEE Communications Magazine, Vol.:56, Issue:11, pp. 110-115. 3. Dolui, K. and Datta, S. K. (2017). Comparison of Edge Computing Implementations: Fog Computing, Cloudlet and Mobile Edge Computing. 2017 Global Internet of Things Summit (GIoTS), Geneva, 2017, pp. 1-6. 4. Bonomi, F., Milito, R., Zhu, J. and Addepalli, S. (2012). Fog computing and its role in the Internet of Things. In Proceedings of the first edition of the MCC workshop on Mobile Cloud Computing. ACM. pp. 13-16. 5. Aazam, M. and Huh, E. N. (2015). E-hamc: Leveraging fog computing for emergency alert service. in 2015 IEEE International Conference on. IEEE Pervasive Computing and Communication Workshops (PerCom Workshops), pp. 518–523. 6. Cao, Y., Chen, S., Hou, P. and Brown, D. (2015). Fast: A Fog Computing assisted distributed analytics system to monitor fall for stroke mitigation. in 2015 IEEE International Conference on Networking, Architecture and Storage (NAS), IEEE, pp. 2–11. 7. Kim, O. T. T. , Tri, N. D., Tran, N. H. and Hong, C. S. (2915). A shared parking model in vehicular network using fog and cloud environment. in 2015 17th Asia-Pacific Network Operations and Management Symposium (APNOMS), IEEE, pp. 321–326. 8. Truong, N. B., Lee, G. M. and Ghamri-Doudane, Y. (2015). Software defined networking-based vehicular adhoc network with fog computing. in 2015 IFIP/IEEE International Symposium on Integrated Network Management (IM), IEEE, pp. 1202–1207. 9. Bastug, E., Bennis, M. and Debbah, M. (2014). Living on the edge: The role of proactive caching in 5g wireless networks. IEEE Communications Magazine, vol. 52, no. 8, pp. 82–89. 10. Pahl, C., Helmer, S., Miori, L., Sanin, J. and Lee, B. (2016). A container- based edge cloud PaaS architecture based on Raspberry pi clusters. in IEEE International Conference on Future Internet of Things and Cloud Workshops (FiCloudW), IEEE, pp. 117–124. 11. Satyanarayanan, M. (2017). The emergence of edge computing. Computer, vol. 50, no. 1, pp. 30–39. 12. Xiao, Y., Simoens, P., Pillai, P., Ha, K. and Satyanarayanan, M. (2013). Lowering the barriers to large-scale mobile crowdsensing. in Proceedings of the 14th Workshop on Mobile Computing Systems and Applications. ACM, p. 9. 13. Satyanarayanan, M. (2015). Edge Analytics in the Internet of Things. IEEE Pervasive Computing, vol. 14, no. 2, pp. 24-31. 14. Xu, X., Huang, S., Feagan, L., Chen, Y., Qiu, Y. and Wang, Y. (2017). EAaaS: Edge Analytics as a Service. 2017 IEEE International Conference on Web Services (ICWS), Honolulu, pp. 349-356. 15. Spice, B. (2018). Carnegie Mellon University, Microsoft join forces to advance Edge Computing Research. News. Retrieved November 22, 2018, from https://www.cmu.edu/news/stories/archives/2018/november/edge-computing-partnership.html 16. Open Edge Computing, Retrieved February 25, 2019, from https://openedgecomputing.org/